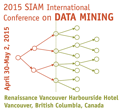
o
Overview, Format
and Audience
o
Paper Submission
o
Invited Speakers
o
Workshop References
o
Previous Edition
of the Workshop
o
Organizers
|
SDM
2015 Workshop on Heterogeneous Learning (Half Day)
|
Overview
by Organizers
The main objective of this workshop is to bring the attention
of researchers to real problems with multiple types of heterogeneities,
ranging from online social media analysis, traffic prediction, to the
manufacturing process, brain image analysis, etc. Some commonly found
heterogeneities include task and domain heterogeneity (as in multi-task
learning, domain adaptation), view heterogeneity (as in multi-view
learning), instance heterogeneity (as in multi-instance learning), label
heterogeneity (as in multi-label learning), oracle heterogeneity (as in
crowdsourcing), etc. In the past years, researchers have proposed various
techniques for modeling a single type of heterogeneity as well as multiple
types of heterogeneities.
This workshop focuses on novel methodologies, applications and
theories for effectively leveraging these heterogeneities. Here we are
facing multiple challenges. To name a few: (1) how can we effectively
exploit the label/example structure to improve the classification
performance; (2) how can we handle the class imbalance problem when facing
one or more types of heterogeneities; (3) how can we improve the
effectiveness and efficiency of existing learning techniques for
large-scale problems, especially when both the data dimensionality and the
number of labels/examples are large; (4) how can we jointly model multiple
types of heterogeneities to maximally improve the classification performance;
(5) how do the underlying assumptions associated with multiple types of
heterogeneities affect the learning methods.
We encourage submissions on a variety of topics, including but
not limited to:
(1) Novel approaches for modeling a single type of heterogeneity,
e.g., task/view/instance/label/oracle heterogeneities.
(2) Novel approaches for simultaneously modeling multiple
types of heterogeneities, e.g., multi-task multi-view learning to leverage
both the task and view heterogeneities.
(3) Novel applications with a single or multiple types of
heterogeneities.
(4) Systematic analysis regarding the relationship between the
assumptions underlying each type of heterogeneity and the performance of
the predictor;
For this workshop, the potential participants and target
audience would be faculty, students and researchers in related areas, e.g.,
multi-task learning, multi-view learning, multi-instance learning,
multi-label learning, etc. We also encourage people with application
background to actively participate in this workshop.
We believe that advancements on these topics will benefit a
variety of application domains.
Format:
The proposed workshop will have keynote talks, invited talks,
oral/poster presentations from paper authors, and panel/open discussions.
Target Audience:
Researchers and practitioners working on problems exhibiting
multiple types of heterogeneity.
|
|
Paper Submission
Return to Top
Key Dates
01/12/2015: Paper
Submission
01/30/2015: Author
Notification
02/09/2015: Camera Ready
Paper Due
Paper Submission Instructions
Papers
submitted to this workshop should be limited to 6 pages formatted using the
SIAM SODA macro (http://www.siam.org/proceedings/macros.php). Authors are required to submit
their papers electronically in PDF format to sdm14hl@gmail.com by 11:59pm MDT, January 12, 2015.
Invited Speakers (Confirmed)
Return to Top
Vipin Kumar (University of Minnesota)
Heng Huang (University of Texas,
Arlington)
Dinggang Shen
(UNC)
Shai Ben-David (University of Waterloo)
Fei Sha (University
of Southern California)
Workshop References
Return to Top
The
proposed workshop builds upon the previous edition at SDM 2014 [1], as well
as other workshops on transfer learning/domain adaptation [2,3,4,5],
crowdsourcing [6,7,8]. The major difference is that, while previous workshops
focused on a single type of heterogeneity, the proposed workshop has a
broader scope, and encourages submissions on modeling both a single and
multiple types of heterogeneities.
Reference
[1]
SDM 2014 Workshop on Heterogeneous Learning
[2]
ICML 2013 Workshop on Theoretically Grounded Transfer Learning
[3]
NIPS 2011 Workshop on Challenges in Learning Hierarchical Models: Transfer
Learning and Optimization
[4]
NIPS 2011 Domain Adaptation Workshop: Theory and Application
[5]
NIPS 2010 Workshop on Transfer Learning Via Rich Generative Models
[6]
ICML 2013 Workshop on Machine Learning Meets Crowdsourcing
[7]
ICML 2012 Workshop on Machine Learning in Human Computation &
Crowdsourcing
[8]
NIPS 2012 Workshop on Human Computation for Science and Computational
Sustainability
Previous Edition of
the Workshop Return to Top
We
organized this workshop at SDM 2014. It consists of 3 keynote talks (1 hour
each), 1 invited talk and 4 paper presentations (half an hour each), and an
open floor discussion (45 minutes). It was well attended throughout the day
with around 25 people.
|
|
Organizers
Return to Top
Organizing Committee
- Jieping
Ye (Arizona State University): jieping.ye@asu.edu
Jieping
Ye (jieping.ye@asu.edu) is an Associate Professor of Computer Science and
Engineering at the Arizona State University. He is a core faculty member of
the Bio-design Institute at ASU. He received his Ph.D. degree in Computer
Science from University of Minnesota, Twin Cities in 2005. His research
interests include machine learning, data mining, and biomedical
informatics. He has served as Senior Program Committee/Area Chair/Program
Committee Vice Chair of many conferences including NIPS, KDD, IJCAI, ICDM,
SDM, ACML, and PAKDD. He serves as an Associate Editor of IEEE Transactions
on Pattern Analysis and Machine Intelligence. He won the SCI Young
Investigator of the Year Award at ASU in 2007, the SCI Researcher of the
Year Award at ASU in 2009, and the NSF CAREER Award in 2010. His papers
have been selected for the outstanding student paper at the International
Conference on Machine Learning in 2004, the KDD best research paper
honorable mention in 2010, the KDD best research paper nomination in 2011
and 2012, the SDM best research paper runner up in 2013, the KDD best
research paper runner up in 2013, and the KDD best student paper award in
2014.
- Yuhong
Guo (Temple University): yuhong@temple.edu
Yuhong
Guo (yuhong@temple.edu) is an Assistant Professor
in the Department of Computer and Information Sciences at Temple
University. She has previously been a Research Fellow at the Australian
National University and a Postdoctoral Fellow at the University of Alberta.
Her research interests include machine learning, natural language
processing, computer vision, bioinformatics and data mining. She has
received the Distinguished Paper Award from the International Joint
Conference on Artificial Intelligence in 2005 and the Outstanding Paper
Award from the AAAI Conference on Artificial Intelligence in 2012. She has
served in program committees of many conferences, including NIPS, ICML,
UAI, AAAI, IJCAI, ACML and SDM.
- Jingrui
He (Arizona State University): jingrui.he@asu.edu
Jingrui He (jingrui.he@asu.edu) is
an Assistant Professor in the School of Computing, Informatics, Decision Systems Engineering at Arizona State
University. She received the Ph.D degree from
School of Computer Science, Carnegie Mellon University in 2010. Her
research interests include rare category analysis and heterogeneous machine
learning with applications in social media analysis, semiconductor
manufacturing, traffic prediction, medical informatics, etc. She has served
on the organizing/senior program committees of many conferences, including
ICML, KDD, IJCAI, ICDM, SDM, etc.
Program Committee
- Xia Ning (NEC Labs America)
- Jianhui
Chen (GE Global Research)
- Jiayu
Zhou (Arizona State University)
- Shuiwang
Ji (Old Dominion University)
- Xinhua
Zhang (National ICT Australia / NICTA)
- Pei
Yang (Arizona State University)
- Hongxia
Yang (IBM Research)
- Yada
Zhu (IBM Research)
|
|